The Case for Human Conversations: Why Data Professionals Remain Critical in the Age of AI Agents Like Gemini in Looker
In Google’s latest Gemini At Work webinar event, the company reviewed AI agents for Looker. Gemini is Google’s AI ecosystem.; Looker is its business intelligence (BI) platform. These artificial intelligence agents are data agents that assist users at each step along the path of transforming data into insights. I am intrigued by Google’s seductive promise of a world where “interacting with your data is as intuitive as your favorite app” and where an AI agent makes “analytics as easy as your favorite app”. (My favorite app is Words with Friends, so this claim sounds to me like a call to have fun with data).
Throughout my 30 years in data analytics, BI and data analytics platforms have striven to make data directly accessible and easy to understand for business users. While AI has made this lofty goal more attainable than ever, I take issue with Google’s implied premise that data professionals, whether data analysts, data scientists, or data engineers, have been key impediments to business users trying to derive insights from their data.
Data Professionals As Part of the Problem
Google points out that “traditional” BI tools have impeded data democratization and slowed the speed to insights because of three key challenges: 1) dependency on IT or data analysts, 2) technical barriers for business users, and 3) traditional BI processes that are slow and hinder agile decision-making. Points 1 and 3 shine a spotlight on data professionals, and point 2 hints at an inability for data professionals to provide proper training and/or user experiences with existing tools.
From what I saw in the Gemini in Looker demo, the data agents do not resolve all these issues for business users. While chatting with data reduces technical barriers, the system as a whole only functions with the direct and active involvement of data professionals. The true good news is that data agents can ease technical burdens for data professionals who in turn can devote their time savings to improving data processes and experiences.
Sorry business users, your dependency on data professionals is far from over…and I claim the need for an on-going relationship should be compelling. For example, while driving vehicles gets easier and easier, most of us still do not want to use our time learning how to build, maintain, or fix our vehicles.
Any experienced data practitioner likely cringes at the thought of letting business users play with data like their favorite app. For example, in a previous job where I built out a data team for a start-up, I confronted a data analytics platform whose core value proposition was providing executives with direct access to data. The resulting structures of the implementation demonstrated the tell-tale signs of the lack of oversight from data professionals. The data model and data flows were ad hoc, data governance was non-existent, and data objects were scattered all over the place. My team and I spent considerable time planning out and implementing fixes and building the relationships with business users that would lead to a healthier data ecosystem.
In other words, the expertise of data professionals exists for a reason. Perhaps ironically, despite the Gemini turbocharge, Looker remains heavily reliant on such expertise to deliver quality experiences for business users.
The Semantic Layer: Data Professionals to the Rescue
Enter the semantic layer. Looker’s semantic layer, built from a flavor of SQL called LookML, is the core engine for delivering reports, dashboards, and insights. This semantic layer is also the hub for creating the end user experience. Google calls the semantic layer the “unsung hero of AI success of the enterprise” because it “ensures consistency, enforces governance, and provides the reliable foundation upon which AI models can be trained.” The semantic layer provides well-governed access to pre-joined views and curated data models. Thus, data professionals remain central to the success of a BI implementation.
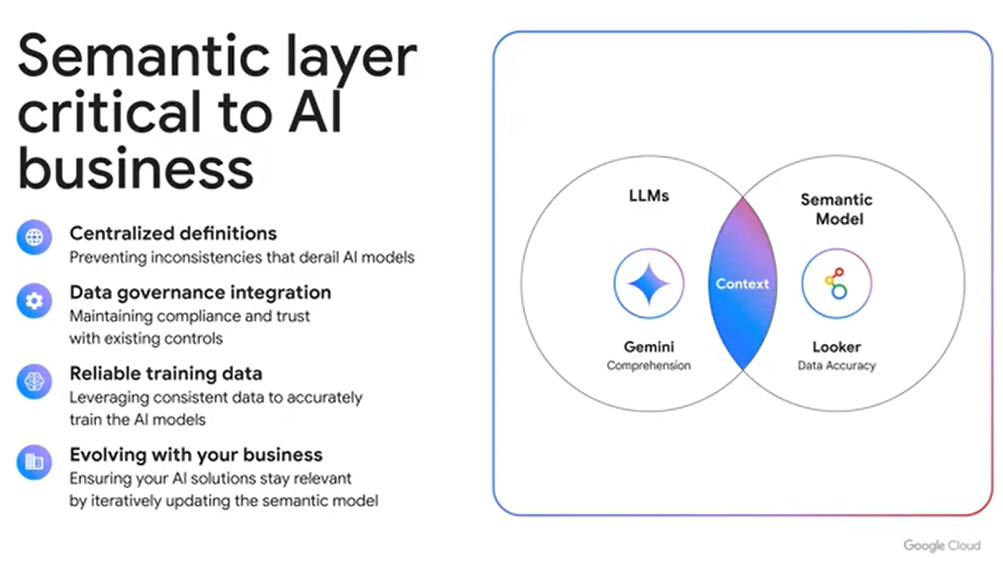
I am a current Looker user and developer, and I am familiar with its functionality and limitations. The demonstration of Looker’s AI agents made me salivate. I can see all the potential time I might save. I can imagine significantly reducing time spent in Google searches, ChatGPT conversations, and poring through support documents. The built-in AI agent is the support document with an ability to carry out requests and implement Looker syntax and features accurately and correctly the first time.
Experienced data professionals can quickly ascend the learning curve of a new BI platform because the good ones are compatible with the basic principles found in what I call “the Insights Supply Chain.” The Insights Supply Chain, when aligned to the organization’s strategy and objectives, efficiently moves data through data functions which transform and package data into data products and insights. Language and syntax is one of the most difficult part of the process of adopting a new platform. The experience can feel like visiting a foreign country knowing exactly what you want and need to do but lacking the words to make things happen in the new environment. The AI agent is the translator, the concierge, and the tour guide. The semantic layer boosted by data professionals empowered with data agents becomes key connective tissue for the Insights Supply Chain.
Conversational Analytics
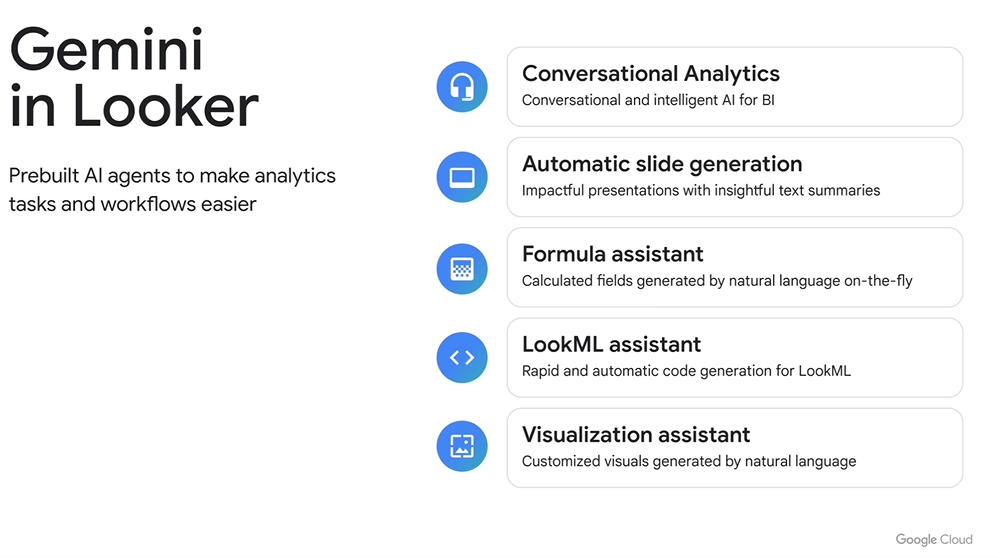
In a well-oiled Looker implementation, the business user never needs to know about the semantic layer. The language of the metrics and dimensions just makes intuitive sense. The well-constructed semantic layer operationalizes Looker’s “conversational analytics”.
The Google Gemini in Looker demo reviews classic use cases like displaying a time series of total monthly revenue. The AI agent accepts a text prompt about the data and provides general descriptions of trends and segments of the data. The AI agent can even disambiguate questions that are malformed or incomplete. This ability to ask questions, whether ad hoc or standardized, gives the business user a lot of freedom to explore data.
However, there is a danger in conducting these conversational analytics in isolation: the potential breakdown of the Insights Supply Chain. A business user might create a conversational “bubble” with the AI agent separate from the world of data professionals. Doing so frays an important feedback loop in the Insights Supply Chain. This breakdown degrades the ability to evolve the data ecosystem for changing business needs and questions.
The back and forth between business users and data professionals that in the moment can feel like a burden is actually important fertilizer for the growth and sustenance of the data ecosystem. Data professionals stay in touch with the business domain and maintains the relevance and utility of the semantic layer. Business users get the opportunity to understand both the limits and the potential for growing the data ecosystem as they collaborate with data professionals. In the ideal case, these collaborations happen in the context of (data) communities of practice. The AI agent cannot replace the communities of practice given its very existence depends on healthy human curation of knowledge.
Promote Human Conversations
If conducted poorly, conversational analytics could actually make the job of data professionals more difficult and, in turn, erode the experience of business users. For example, when data professionals build dashboards, they know the finite set of ways a business user can view and use the data. The finite use cases facilitate the anticipation of questions and challenges. Data professionals have full context when receiving feedback on the dashboard experience. The conversational analytics with AI agents are essentially unconstrained. Business users can navigate unguided in unanticipated directions and misinterpret data and relationships along the way. Debugging the business user’s experience could require reconstructing whole dialogues and trains of thought.
A privacy-friendly logging system could partially mediate the problem of unbounded conversational analytics. A data professional could periodically review these logs (or even unleash other AIs to review, summarize, and synthesize these conversations) and flag problematic areas for addressing with the business user. Yet, I imagine reviewing these logs in isolation would be a lot more burdensome than the back and forth in conventional BI processes. Thus, I propose that AI agents should promote human conversations as a core feature and mission.
AI agents in the data ecosystem should plug into a broad knowledge management framework or platform. These agents would know the limits of their understanding and alert a business user to the need to converse with a particular data expert. Business users should proactively schedule reviews of their findings and sessions with data professionals. Business users must avoid getting comfortable in a self-nurtured bubble with AI agents. Looker data agents that help build presentation decks should automatically share these data products in a knowledge management repository. Data professionals should proactively seek feedback on a periodic basis and even field test AI agents with a range of questions. A community of practice would continually motivate these activities by reinforcing the value proposition of human conversations.
Conclusion
AI agents, data agents, operating in a data ecosystem represent an important advancement. They offer the potential to save time, effort, and money. These savings should be directly invested in the data professionals who will remain central to maintaining the health of the data ecosystem through tools like the semantic layer. With more time and better utilized resources, data professionals can enable business users to get to insights faster, they can focus on more advanced analytics, they can improve data processes with more effective human conversations, and, importantly, they can strengthen the foundations of a data community of practice.
Follow Ahan Analytics, LLC on Facebook and get notifications for the next analytic thoughts!